Insurance
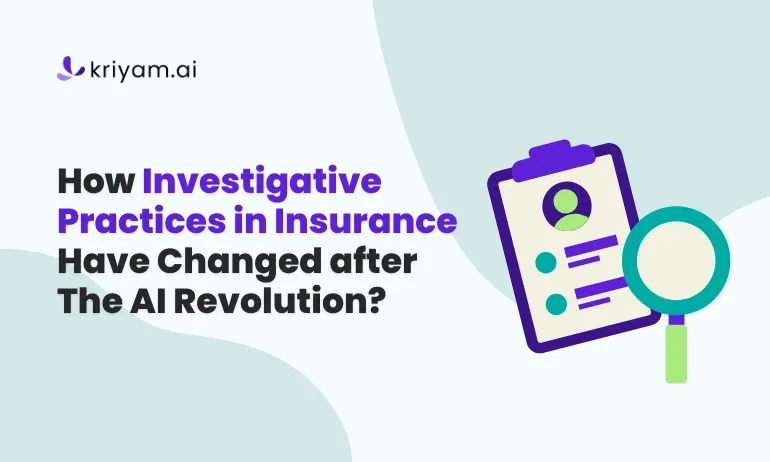
How Investigative Practices in Insurance have Changed after the AI Revolution?
Fraud is one of the most significant challenges within the insurance industry, resulting in loss of billions of rupees each year in India and increasing premiums for honest buyers. Prior to usage of AI, inquiries were reactive, labor-intensive, and manual. Fraud detection depended on human judgments, paper files, and drawn-out verification exercises, making it simple for fraudulent parties to capitalize on inefficiencies within the system.
Following AI, fraud detection turned proactive and data-driven. AI-based systems examine huge datasets in real time and identify suspicious claims immediately. Automatic identity verification, document authentication, and predictive analysis minimize errors, accelerate investigations, and improve accuracy. As fraud continues to develop, AI-driven solutions are now a must for insurers to be ahead of the game and keep the system fair and efficient.
Challenges in Insurance Fraud Investigations
Insurance companies in India are challenged by the poor efficiency of the whole investigative procedures. But the new schemes of fraud are becoming so sophisticated and complex that they are getting ahead of the fraud control units, which themselves still use outmoded manual practices and legacy systems. Their practices fail to ensure timely responses and decrease overall effectiveness. Some of the leading causes of increased difficulty doing fraud investigation include:
Heavy Reliance on Manual Processes: The laborious process of documenting and paperwork used in investigations usually slows down the whole process. In fact, sharing the data based on spreadsheets or messaging platforms allows for various errors and complications in the workflow.
Limited Transparency and Tracking: Generally, insurers don't have tracking and visibility in the investigation in real time; therefore, in between gaps of the investigations, frauds could be hidden. This lack of oversight enables fraudulent activities to easily slip past the system.
Complexities in Vendor Collaboration: Poor channels of communication with external surveyors and investigators lead to data collection efforts not being aligned. This, unfortunately, could enhance the turnaround time by a great amount.
Data Consolidation Difficulties: Usually, the case history details are scattered all across different systems, which do not communicate with each other very well. This leads to a siloed approach, and thus it becomes very difficult to track and monitor the repeated fraudulent behaviors.
Lack of Data Privacy: The absence of data privacy exposes sensitive data of policyholders and investigations to being known by unauthorized persons through current investigations, thus raising fears of their possible misuse, legal penalties, and loss of customer trust. To add insult to injury, these very limitations render investigation processes inefficient and prone to error.
The gaps present in the investigation processes delay the investigations and provide opportunities for possible fraudsters to take advantage of the system vulnerabilities. Therefore, there is a need for the industry to adopt smarter and streamlined processes of investigations to tackle these issues.
Read Also: Fraud Risk Mitigation Strategies To Protect Your Insurance Business
The AI Advantage: How AI is Revolutionizing Insurance Fraud Investigations?
Insurance fraud investigations have historically been time-consuming, based on slow, labor-intensive, and error-prone manual processes. With the use of AI, investigations are now more accurate, data-driven, and highly automated. Below, we contrast each phase of an insurance investigation, illustrating how AI has transformed the process with real-world examples.
The following components form part of traditional investigative practices in insurance:
Initial Fraud Detection: From Manual Red Flags to AI-Driven Risk Assessment Detectives used their expertise and pre-programmed rules to detect fraudulent claims. They would manually review for red flags like inflated damages, repeated claims by the same individual, or conflicting statements. But this process was time-consuming and frequently failed to detect sophisticated fraud schemes. AI-based fraud detection systems scan large volumes of data in real-time, identifying patterns that are likely to be fraud. AI calculates fraud risk score for every claim based on factors such as claim history, behavioral anomalies, and cross-checking with prior fraud cases. For example: If someone reports a stolen vehicle. Previously, investigators would review police reports and interview witnesses. Today, AI can scan databases in an instant, review GPS records, and cross-check the claimant's previous claims. If it detects that the individual has made similar claims repeatedly or that the GPS indicates the vehicle was in motion after the theft was reported, the case is marked for investigation.
Evidence Gathering: From Manual Document Verification to AI-Based Validation Detectives manually gathered medical reports, bills, and police reports, confirming them via phone calls or on-site visits. Scammers were able to create fake documents, and it was hard to identify discrepancies. AI verifies documents automatically with Optical Character Recognition (OCR) and blockchain authentication. It is able to identify changes, match invoices to actual transactions, and authenticate records against official databases in seconds. For example: A claimant files a hospital bill for an injury. AI reads the document and cross-verifies it with the hospital's official records. If it finds different fonts, conflicting timestamps, or a record that does not exist in the hospital's system, the claim is marked as possibly fraudulent.
Identity Verification: From Simple ID Checks to Biometric Authentication Insurance providers based their identity verification on conventional checks such as name, address, and ID number. Fraudsters could make false or stolen identities in order to make claims. AI-based identity verification integrates facial recognition, biometric identification, and real-time data verification. AI guarantees that the claimant is the true policyholder, thus curbing identity fraud. For example: A scammer tries to make a claim with a stolen ID. AI demands a live video confirmation where the claimant must make certain movements, like blinking or tilting their head. If the live video doesn't match the ID photo, the system automatically rejects the claim.
Incident Analysis: From Manual Investigations to AI-Powered Pattern Recognition Traditionally, investigators had to read reports by hand, contrast them with historical cases, and search for discrepancies. This was slow and generally did not identify organized fraud rings. AI examines claim information, medical files, and external sources such as social media and GPS tracking. It identifies anomalies, contrasts claims with historical cases of fraud, and flags inconsistencies. For example: An individual submits a claim for disability, claiming they cannot work because of a back injury. AI goes through their social media and discovers a recent video of them running in a marathon. The system automatically flags the claim for closer examination, weeks of labor-intensive investigation saved.
Surveillance and Monitoring: From Physical Surveillance to AI-Driven Digital Tracking Private investigators were hired by insurance companies to track suspicious claimants suspected of fraud. This was expensive, used lots of manpower, and yielded little evidence. AI tracks social media usage, spending habits, and location information to identify suspicious activity. Sophisticated AI algorithms scrutinize the actions of claimants and cross-check them with their stated injuries or accidents. For example: An individual reports they had a serious leg injury, rendering them incapable of working. AI cross-checks their fitness tracker history, where they've been running every day. AI also finds recent payments to an agency offering travel services, implying they purchased a hiking trip. The system identifies the claim as fraudulent, saving the expense of deploying expensive physical surveillance.
Reporting and Decision Making: From Manual Report Compilation to AI-Generated Insights Detectives manually prepared case reports, outlining results and conclusions. This was done over weeks and was subject to inconsistencies. AI generates automated, structured reports, such as fraud probability scores, crucial evidence, and recommended action. AI-based case summaries enable insurers to make fast, data-driven decisions. For example: Rather than wasting hours building a report, an investigator gets an AI-written summary with all the suspicious features, including timestamps, document authentication results, and fraud flags. The insurer can thus accept or reject the claim in a matter of minutes.
AI has revolutionized insurance fraud investigations from slow manual affairs to fast, data-driven, and highly accurate systems. With the adoption of AI, insurers are able to:
- Identify fraud in real-time with pattern analysis
- Minimize false positives, allowing for speedy processing of authentic claims
- Eradicate paperwork and human errors
- Stop fraud before claims are paid, saving millions in losses
With AI to examine data, identify inconsistencies, and streamline verification, insurance providers can guarantee an easier, more equitable, and more consistent claims process to both insurers and policyholders.
Read Also: AI in Insurance Claims: 7 Key Transformative Impacts
How Latest Technologies Help in Insurance Investigations?
Unlike old-school techniques, technology of recent times is completely transforming investigative practices in insurance. They offer solutions that optimize on already provided efficiency and performance. Key technologies revolutionizing investigative practices include:
Digitalization and Automation of Investigation: The transition to digitized processes and automation has substantially enhanced insurance investigations. Automated fraud detection systems, real-time tracking, and digital forensics assist insurers in streamlining workflow, contain less manual work, and provide more accuracy. These technologies enhance the speed of fraud detection and monitoring, digital processing of the data, and security in investigations.
Document Verification: Because of advanced technologies, document verification becomes simple and fast and at the same time allows the investigators to prove the authenticity of a document. Tools examining and scanning documents for forgery indicators greatly minimize risks associated with manual document verification.
Identity verification: With identity verification tools, the insurer ensures that a customer is who they say they are, thus preventing identity theft and fraudulent claims from happening. Automated systems can flag any discrepancies between the information provided against various databases, so while the verification may seem more formidable, it is very reliable.
Artificial intelligence (AI) claims verification: AI has changed the way insurance companies detect fraud and administer claims, allowing insurers to analyze large volumes of structured and unstructured data to identify unusual patterns, build red flags for claims needing further investigation, and make better decisions about claims. Some of the areas where AI impacts the claims verification process are:
- Tariff Check & Overbilling Alerts: AI compares claims for medical, auto repair, and other services against standard tariffs to flag unreasonable or excessive charges. A medical provider who bills significantly higher than the average charge for a specific procedure will automatically trigger a flag showing excessive billing.
- Unnecessary or Exaggerated Medical Bills: AI cross-checks treatments against treatment documented and the standardized treatment for similar diagnosis. When a claim for care includes excessive or unnecessary treatment or exaggerations on injuries, it will be flagged for review by the process.
- Duplicate Claims Review: AI can cross-check multiple databases in real time to identify similarities in claims for the same event. This knowledge is critical for detecting double-dipping fraud, which refers to submitting claims for reimbursement to multiple insurance providers.
- Staged Accidents: AI analyzes additional variables, such as prior claims, the type of vehicle damaged, and location data to get a variety of angles for comparison to determine staged accidents. Moreover, AI can identify organized fraud rings, including groups of people who engage in excessive claims or false accidents to file repeated claims for reimbursement from the insurer.
- Policyholder Behavioral Analysis: AI monitors behavioral trends and recognizes anomalies, for example: if a policyholder files multiple, high-value claims soon after purchasing a policy. Patterns like these are often red flags for fraud.
Utilizing AI helps insurers streamline claims processing, reduce fraudulent payouts, and drive efficiency so that legitimate claims are paid quickly and fraudulent ones stop in their tracks.
Read Also: How AI is redefining insurance in 2025?
Using AI and Automation for Enhanced Investigation Efficiency
For the enhancement of fraud management efficiency, the application of advanced technology-like AI is not only generally but also nearly universally accepted by everybody. AI has already proven its value when applied to insurance fraud detection. The aid of AI systems provides insurance companies with the access to identify, prevent, and respond to fraud in an intelligent manner, saving time, resources, and financial investment.
Kriyam.ai Investigation Platform is an excellent example of how firms can use AI to augment their investigation processes. This leads to a far more effective workflow through automated composite processes and smart technology. Kriyam.ai is changing the investigation process in the following ways:
- Paperless Operations Centralized Case Monitoring
- AI-Powered Workflows
- Efficient Vendor Coordination
- Data Protection
Kriyam.ai, through its implementation, has seen an increased accuracy, less error, and high productivity within its field operations. It is helping the insurers by shortening the time needed to complete the case resolution during pre-issuance and claims processes with a median cut of 25% on investigation times. Investigators also find themselves saving over 12 man-hours a week otherwise spent doing manual data entries and reporting, allowing them to concentrate on more tedious investigations that involve cross-checks.
Final Thoughts
In essence, effective investigative practices in insurance sector are important in the insurance industry due to the rise in frauds as per the insurance industry trends in 2024. Investigation best practices could involve traditional methods and modern technological solutions. Advanced technologies can help the insurance industry to raise productivity, effectiveness, and client trust.
Insurers can adopt these advanced technologies for improving efficiency, effectiveness, and trust among the policyholders by investing in AI-enabled investigation solutions and utilizing automation to cut down on fraud. Investigative practices in insurance sector remain resilient against fraud and better serve consumers. The technology for better investigation is the key to the future of insurance.
Frequently Asked Questions [FAQs]
1. What triggers an insurance fraud investigation?
An insurance fraud investigation can start due to suspicious activities, discrepancies in claims, or patterns suggesting fraud.
2. What steps are involved in an insurance fraud investigation?
The steps generally include initial detection, evidence gathering, identity verification, and report compilation.
3. Can insurance fraud be hard or soft?
Yes, fraud can take forms such as hard fraud, where claims are entirely fabricated, and soft fraud, where legitimate claims are inflated.
4. Who investigates insurance fraud?
Fraud investigations are conducted by in-house fraud teams, law enforcement, or third-party firms specializing in fraud detection.
5. What are common types of insurance fraud?
Common types include false claims, staged accidents, inflated damage claims, and identity theft.
6. How can technology aid in detecting fraud?
Technology enhances fraud detection through automated analysis, real-time verification, and advanced data analytics.

Kriyam.ai Content Team
30th March, 2025
Latest
LATEST BLOGS
Technology
Top 5 Best Field Service Management Software in 2025
Best Field Service Management Software

Shreyas R
27th November, 2023
FEATURED
Technology
Top 5 Best Field Service Management Software in 2025
Best Field Service Management Software

Shreyas R
27th November, 2023